Monitor Confluent Cloud Clients¶
When developing Apache Kafka® applications on Confluent Cloud, it is important to monitor the health of your deployment to maintain optimal performance. Performance monitoring helps to achieve this by providing a quantitative account of the health of each Kafka or Confluent component.
Since production environments are dynamic–that is, data profiles may change, you may add new clients and enable new features–performance monitoring is crucial. Ongoing monitoring is as much about identifying and responding to potential failures as it is about ensuring the service goals are consistently met even as the production environment changes. Before going into production, you should have a robust monitoring system for all the producers, consumers, topics, and any other Kafka or Confluent components you’re using.
The information on this page helps you configure a robust performance monitoring system when working with Confluent Cloud.
Monitor clients on the Confluent Cloud Console¶
The Clients overview page in Confluent Cloud organizes the clients into the principal groups. You can view the client metrics that are aggregated within a principal. You can also filter for unsupported client versions.
To view and monitor the installed clients on the Clients overview page of the Confluent Cloud cluster:
On the Confluent Cloud Console, browse to the environment and the cluster, and click Clients.
For a specific principal, click the Producers, Consumers, or Consumer lag tabs to see applicable clients in the category.
For each principal, aggregate client metrics are displayed.
To see consumer lag information, click the Consumer lag tab.
Consumer lag refers to the delay between the production and consumption of messages in Kafka, which can significantly impact your system’s overall performance.
For details about monitoring consumer lag, see Monitor Kafka Consumer Lag in Confluent Cloud.
Expand one or all principals to see clients for the principal.
For each producer client, the following metric is displayed:
Average request latency
This is the average latency between the client and the Kafka cluster.
This metric is only available for the clients using Java 3.8+ when the client configuration,
enable.metrics.push
, is set totrue
(the default setting).In the initial release, this metric is only available on AWS, for the us-west-2 region.
For the best practices in the latency monitoring in Confluent Cloud, refer to Optimize Confluent Cloud Clients for Latency.
Monitor unsupported client versions¶
On the Clients overview page of the Confluent Cloud Console, you can check if you are using an unsupported client version.
It is recommended that you update your client versions regularly to avoid undesired behavior in your clusters. Some issues that can arise from running unsupported client versions are:
- Security vulnerabilities: Confluent Cloud is regularly updated with security patches and bug fixes. Using an unsupported client version means that you may be missing critical security updates, leaving your applications and data vulnerable to potential security threats or exploits.
- Lack of support: Confluent provides official support and maintenance for supported client versions only. If you encounter issues or bugs while using an unsupported client version, Confluent may not be able to provide assistance or troubleshooting help, leaving you to resolve the issues on your own.
- Missing features and improvements: Newer versions of client libraries often introduce new features, performance improvements, and bug fixes.
- Potential service disruptions: Confluent Cloud may introduce changes or updates that are designed to work with supported client versions. Using an unsupported client version could lead to service disruptions or unexpected behavior when such changes are made.
To ensure a stable, secure, and well-supported experience with Confluent Cloud, it is highly recommended that you use the officially supported client versions for your specific programming language or framework. See the version supportability guide for the supported client versions and their compatibility with Confluent Cloud services and features.
To monitor clients versions on the Confluent Cloud Console:
Select your cluster name.
Click Clients, and navigate to the Producer or Consumer tab depending on which clients you would like to view.
Select Show clients with traffic only to filter down to only clients that have produced/consumed a message in the last 10 minutes.
Principals containing unsupported clients are indicated by a yellow warning icon.
Click the dropdown arrow to view unsupported clients associated with this principal.
Supported versions are indicated with a green status, and the unsupported versions are indicated with a yellow status.
Locate the unsupported version and update the client.
Some clients report “unknown” as the version because those clients do not emit the ApiVersion.
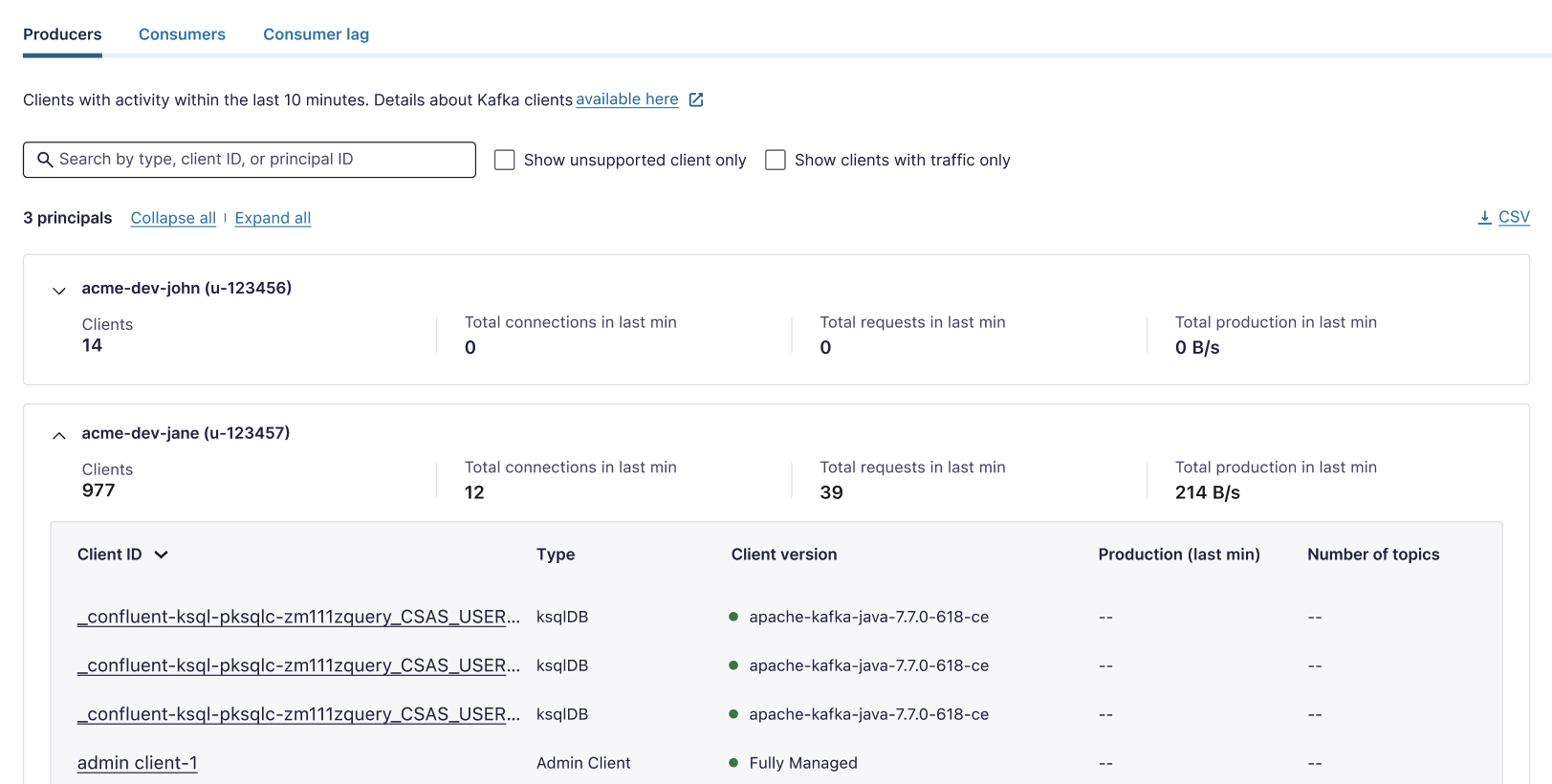
Metrics API¶
The Confluent Cloud Metrics provides programmatic access to actionable metrics for your Confluent Cloud deployment, including server-side metrics for the Confluent-managed services. However, the Metrics API does not allow you to get client-side metrics. To retrieve client-side metrics, see Producers and Consumers.
The Metrics API, enabled by default, aggregates metrics at the topic and cluster level. Any authorized user can gain access to the metrics that allow you to monitor overall usage and performance. To get started with the Metrics API, see the Confluent Cloud Metrics documentation.
You can use the Metrics API to query metrics at the following granularities (other resolutions are available if needed):
- Bytes produced per minute grouped by topic
- Bytes consumed per minute grouped by topic
- Max retained bytes per hour over two hours for a given topic
- Max retained bytes per hour over two hours for a given cluster
You can retrieve the metrics easily over the internet using HTTPS, capturing them at regular intervals to get a time series and an operational view of cluster performance. You can integrate the metrics into any cloud provider monitoring tools like Azure Monitor, Google Cloud’s operations suite (formerly Stackdriver), or Amazon CloudWatch, or into existing monitoring systems like Prometheus and Datadog, and then plot them in a time series graph to see usage over time. When writing your own application to use the Metrics API, see the full API specification to use advanced features.
Client JMX metrics¶
Kafka applications expose some internal Java Management Extensions (JMX) metrics,
and many users run JMX exporters to feed the metrics into their monitoring
systems. You can retrieve JMX metrics for your client applications and the
services you manage (though not for the Confluent-managed services, which are
not directly exposed to users) by starting your Kafka client applications with
the JMX_PORT
environment variable configured. There are many
Kafka-internal metrics that are exposed through JMX to
provide insight on the performance of your applications.
Producers¶
Throttling¶
Depending on your Confluent Cloud service plan, you may be limited to certain throughput rates for produce (write). If your client applications exceed the produce rates, the quotas on the brokers detect it and the brokers throttle the client application requests. It’s important to ensure your applications aren’t consuming more resources than they should be. If the brokers throttle the client application requests, consider the following two options:
- Make modifications to the application to optimize its throughput. For more information on how to optimize throughput, see Optimize Confluent Cloud Clients for Throughput.
- Choose a cluster configuration with higher limits. In Confluent Cloud, you can choose from Standard and Dedicated clusters (customizable for higher limits). The Metrics API can give you some indication of throughput from the server side, but it doesn’t provide throughput metrics on the client side. For more information about cluster limits, see Kafka Cluster Types in Confluent Cloud.
To get throttling metrics per producer, monitor the following client JMX metrics:
Metric | Description |
---|---|
kafka.producer:type=producer-metrics,client-id=([-.w]+),name=produce-throttle-time-avg |
The average time in ms that a request was throttled by a broker |
kafka.producer:type=producer-metrics,client-id=([-.w]+),name=produce-throttle-time-max |
The maximum time in ms that a request was throttled by a broker |
User processes¶
To further tune the performance of your producer, monitor the producer time
spent in user processes if the producer has non-blocking code to send messages.
Using the following io-ratio
and io-wait-ratio
metrics where user
processing time is the fraction of time not spent in either of these. If time in
these are low, then the user processing time may be high, which keeps the single
producer I/O thread busy. For example, you can check if the producer is using
any callbacks, which are invoked when messages have been acknowledged and run in
the I/O thread:
Metric | Description |
---|---|
kafka.producer:type=producer-metrics,client-id=([-.w]+),name=io-ratio |
Fraction of time that the I/O thread spent doing I/O |
kafka.producer:type=producer-metrics,client-id=([-.w]+),name=io-wait-ratio |
Fraction of time that the I/O thread spent waiting |
Consumers¶
Throttling¶
As mentioned earlier with producers, depending on your Confluent Cloud service plan, you may be limited to certain throughput rates for consume (read). If your client applications exceed these consume rates, consider the two options mentioned earlier.
To get throttling metrics per consumer, monitor the following client JMX metrics:
Metric | Description |
---|---|
kafka.consumer:type=consumer-fetch-manager-metrics,client-id=([-.w]+),name=fetch-throttle-time-avg |
The average time in ms that a broker spent throttling a fetch request |
kafka.consumer:type=consumer-fetch-manager-metrics,client-id=([-.w]+),name=fetch-throttle-time-max |
The maximum time in ms that a broker spent throttling a fetch request |
Consumer lag¶
Your application’s consumer lag
is the number of records for any partition
that the consumer is behind in the log. This metric is particularly important
for real-time consumer applications where the consumer should be processing the
newest messages with as low latency as possible.
Monitor consumer lag to see whether the consumer is able to fetch records fast enough from the brokers.
Also consider how the offsets are committed. For example, exactly-once semantics
(EOS) provide stronger guarantees while potentially increasing consumer lag. If
you are capturing JMX metrics, you can monitor records-lag-max
:
Metric | Description |
---|---|
kafka.consumer:type=consumer-fetch-manager-metrics,client-id=([-.w]+),records-lag-max |
The maximum lag in terms of number of records for any partition in this window. An increasing value over time is your best indication that the consumer group isn’t keeping up with the producers. |
Client librdkafka metrics¶
The librdkafka library provides advanced Kafka telemetry that can be used to monitor the performance of Kafka producers and consumers. Similar to JMX metrics, you can configure the librdkafka library to emit internal metrics at regular intervals, which can then be fed into your monitoring systems. While JMX metrics provide high-level statistics for Kafka consumers and producers, the librdkafka metrics provide telemetry at the broker and topic-partition level. For more information, see statistics metrics in the librdkafka documentation.
...
"throttle": {
"min": 0,
"max": 0,
"avg": 0,
"sum": 0,
"stddev": 0,
"p50": 0,
"p75": 0,
"p90": 0,
"p95": 0,
"p99": 0,
"p99_99": 0,
"outofrange": 0,
"hdrsize": 17520,
"cnt": 0
}
...
Metric | Description |
---|---|
throttle |
Top level object that contains rolling window statistics for broker throttling in milliseconds. |
min |
The smallest value. |
max |
The largest value. |
avg |
The average value. |
sum |
The sum of values. |
stddev |
The standard deviation (based on histogram). |
p50 |
50th percentile. |
p75 |
75th percentile. |
p90 |
90th percentile. |
p99 |
99th percentile. |
p99_99 |
99.99th percentile. |
outofrange |
Values skipped due to out of histogram range. |
hdrsize |
Memory size of Hdr Histogram. |
cnt |
Number of values sampled. |