Stream Processing Concepts in Confluent Cloud for Apache Flink¶
Apache Flink® SQL, a high-level API powered by Confluent Cloud for Apache Flink, offers a simple and easy way to leverage the power of stream processing. With support for a wide variety of built-in functions, queries, and statements, Flink SQL provides real-time insights into streaming data. Time is a critical element in stream processing, and Flink SQL makes it easy to process data as it arrives, avoiding delays. By using SQL syntax, you can declare expressions that filter, aggregate, route, and mutate streams of data, simplifying your data processing workflows.
Stream processing¶
Streams are the de-facto way to create data. Whether the data comprises events from web servers, trades from a stock exchange, or sensor readings from a machine on a factory floor, data is created as part of a stream.
When you analyze data, you can either organize your processing around
bounded
or unbounded
streams, and which of these paradigms you choose
has significant consequences.
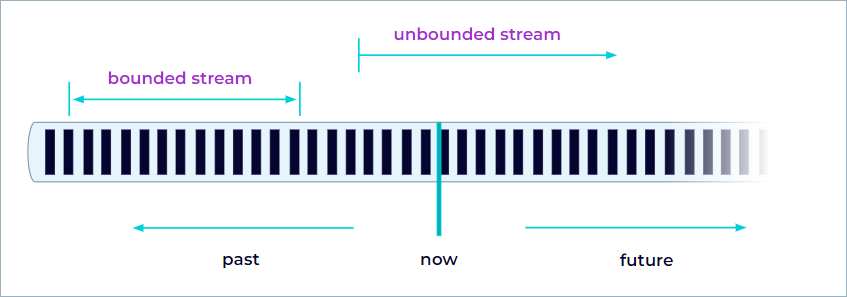
Batch processing is the paradigm at work when you process a bounded data stream. In this mode of operation, you can choose to ingest the entire dataset before producing any results, which means that it’s possible, for example, to sort the data, compute global statistics, or produce a final report that summarizes all of the input.
Stream processing, on the other hand, involves unbounded data streams. Conceptually, at least, the input may never end, and so you must continuously process the data as it arrives.
A Confluent Cloud for Apache Flink application can consume real-time data from streaming sources like message queues or distributed logs, like Apache Kafka®. But Flink can also consume bounded, historic data from a variety of data sources. Similarly, the streams of results being produced by a Flink application can be sent to a wide variety of systems that can be connected as sinks.
Parallel dataflows¶
Programs in Flink are inherently parallel and distributed. During execution, a stream has one or more stream partitions, and each operator has one or more operator subtasks. The operator subtasks are independent of one another, and execute in different threads and possibly on different machines or containers.
The number of operator subtasks is the parallelism of that particular operator. Different operators of the same program may have different levels of parallelism.
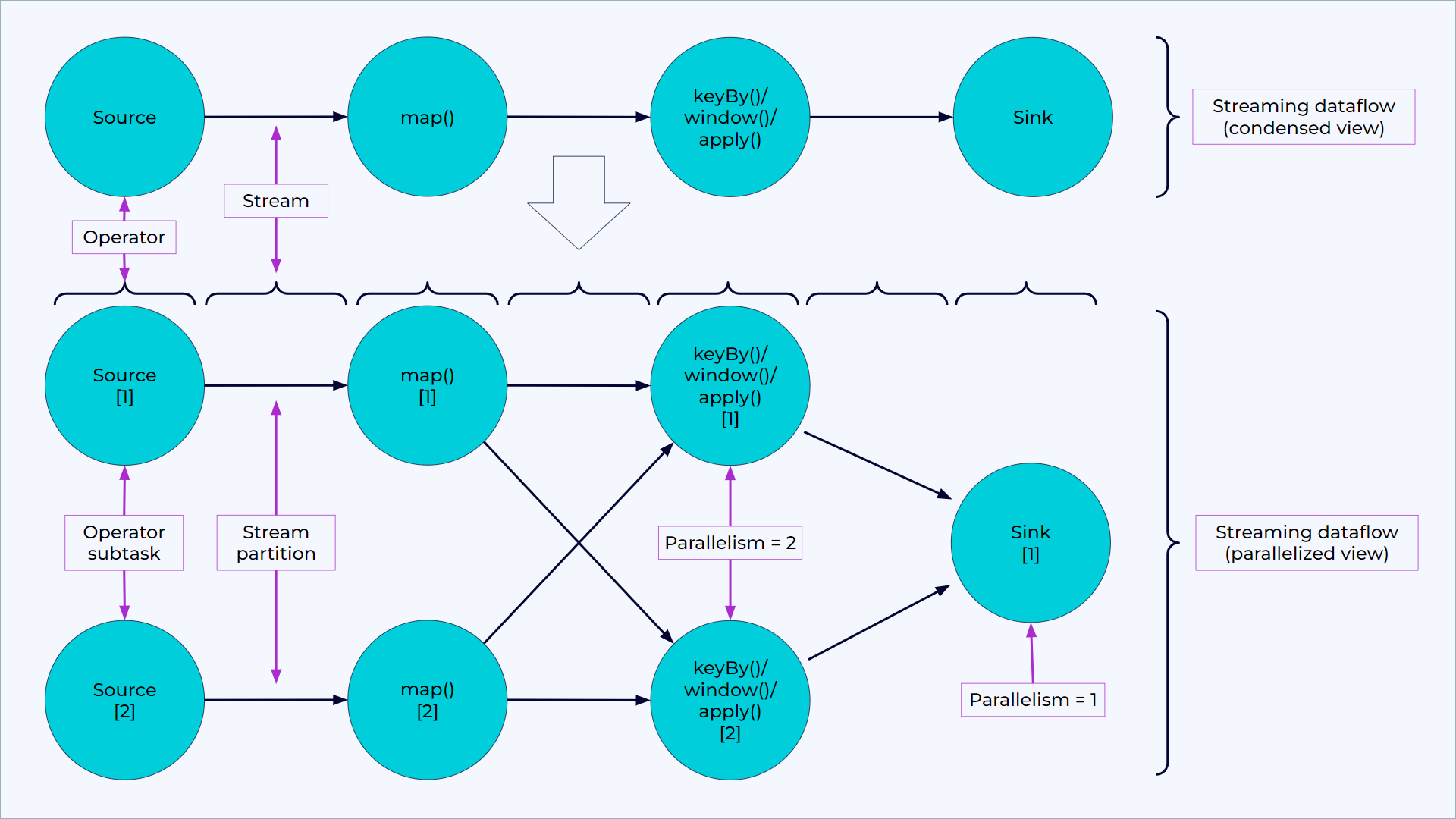
A parallel dataflow in Flink with condensed view (above) and parallelized view (below).¶
Streams can transport data between two operators in a one-to-one (or forwarding) pattern, or in a redistributing pattern:
- One-to-one streams (for example between the Source and the map() operators in the figure above) preserve the partitioning and ordering of the elements. That means that subtask[1] of the map() operator will see the same elements in the same order as they were produced by subtask[1] of the Source operator.
- Redistributing streams (as between map() and keyBy/window above, as well as between keyBy/window and Sink) change the partitioning of streams. Each operator subtask sends data to different target subtasks, depending on the selected transformation. Examples are keyBy() (which re-partitions by hashing the key), broadcast(), or rebalance() (which re-partitions randomly). In a redistributing exchange the ordering among the elements is only preserved within each pair of sending and receiving subtasks (for example, subtask[1] of map() and subtask[2] of keyBy/window). So, for example, the redistribution between the keyBy/window and the Sink operators shown above introduces non-determinism regarding the order in which the aggregated results for different keys arrive at the Sink.
Timely stream processing¶
For most streaming applications it is very valuable to be able re-process historic data with the same code that is used to process live data - and to produce deterministic, consistent results, regardless.
It can also be crucial to pay attention to the order in which events occurred, rather than the order in which they are delivered for processing, and to be able to reason about when a set of events is (or should be) complete. For example, consider the set of events involved in an e-commerce transaction, or financial trade.
These requirements for timely stream processing can be met by using event time timestamps that are recorded in the data stream, rather than using the clocks of the machines processing the data.
Stateful stream processing¶
Flink operations can be stateful. This means that how one event is handled can depend on the accumulated effect of all the events that came before it. State may be used for something simple, such as counting events per minute to display on a dashboard, or for something more complex, such as computing features for a fraud detection model.
A Flink application is run in parallel on a distributed cluster. The various parallel instances of a given operator will execute independently, in separate threads, and in general will be running on different machines.
The set of parallel instances of a stateful operator is effectively a sharded key-value store. Each parallel instance is responsible for handling events for a specific group of keys, and the state for those keys is kept locally.
The following diagram shows a job running with a parallelism of two across the first three operators in the job graph, terminating in a sink that has a parallelism of one. The third operator is stateful, and a fully-connected network shuffle is occurring between the second and third operators. This is being done to partition the stream by some key, so that all of the events that need to be processed together will be.
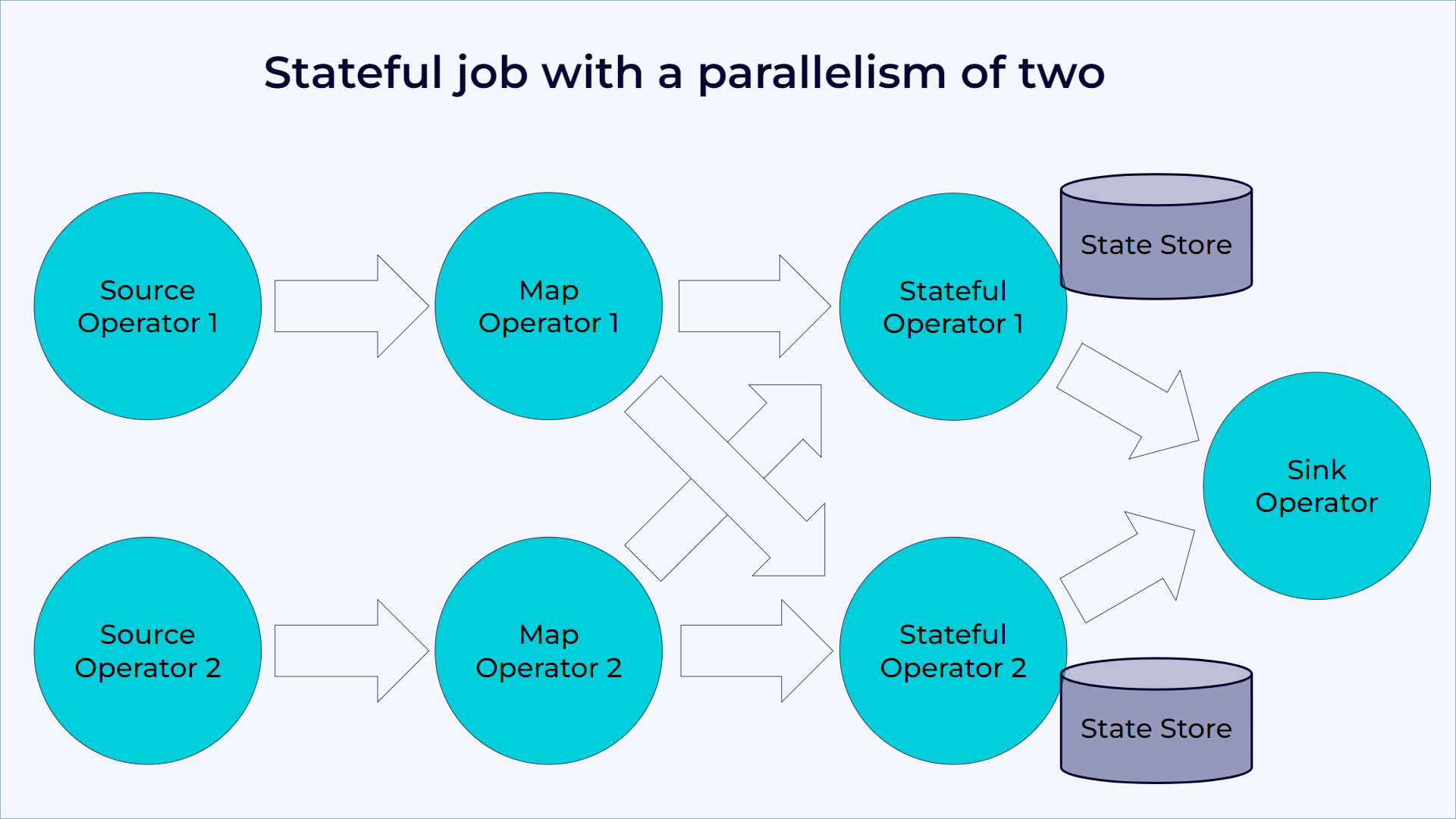
A Flink job running with a parallelism of two.¶
State is always accessed locally, which helps Flink applications achieve high throughput and low-latency.
State management¶
Fault tolerance via state snapshots¶
Flink is able to provide fault-tolerant, exactly-once semantics through a combination of state snapshots and stream replay. These snapshots capture the entire state of the distributed pipeline, recording offsets into the input queues as well as the state throughout the job graph that has resulted from having ingested the data up to that point. When a failure occurs, the sources are rewound, the state is restored, and processing is resumed. As depicted above, these state snapshots are captured asynchronously, without impeding the ongoing processing.
Table programs that run in streaming mode leverage all capabilities of Flink as a stateful stream processor.
In particular, a table program can be configured with a state backend and various checkpointing options for handling different requirements regarding state size and fault tolerance. It is possible to take a savepoint of a running Table API and SQL pipeline and to restore the application’s state at a later point in time.
State usage¶
Due to the declarative nature of Table API & SQL programs, it is not always obvious where and how much state is used within a pipeline. The planner decides whether state is necessary to compute a correct result. A pipeline is optimized to claim as little state as possible given the current set of optimizer rules.
Conceptually, source tables are never kept entirely in state. An implementer deals with logical tables, named Tables and Topics. Their state requirements depend on the operations that are in use.
Queries such as SELECT ... FROM ... WHERE
which consist only of
field projections or filters are usually stateless pipelines. But operations
like joins, aggregations, or deduplications require keeping intermediate
results in a fault-tolerant storage for which Flink state abstractions are used.
Refer to the individual operator documentation for more details about how much state is required and how to limit a potentially ever-growing state size.
For example, a regular SQL join of two tables requires the operator to keep both input tables in state entirely. For correct SQL semantics, the runtime needs to assume that a match could occur at any point in time from both sides of the join. Flink provides optimized window and interval joins that aim to keep the state size small by exploiting the concept of watermark strategies.
Another example is the following query that computes the number of clicks per session.
SELECT sessionId, COUNT(*) FROM clicks GROUP BY sessionId;
The sessionId
attribute is used as a grouping key and the continuous
query maintains a count for each sessionId
it observes. The
sessionId
attribute is evolving over time and sessionId
values
are only active until the session ends, i.e., for a limited period of
time. However, the continuous query cannot know about this property of
sessionId
and expects that every sessionId
value can occur at
any point of time. It maintains a count for each observed sessionId
value. Consequently, the total state size of the query is continuously
growing as more and more sessionId
values are observed.
Dataflow Model¶
Flink implements many techniques from the Dataflow Model. The following articles provide a good introduction to event time and watermark strategies.
- Blog post: Streaming 101 by Tyler Akidau
- Dataflow Model